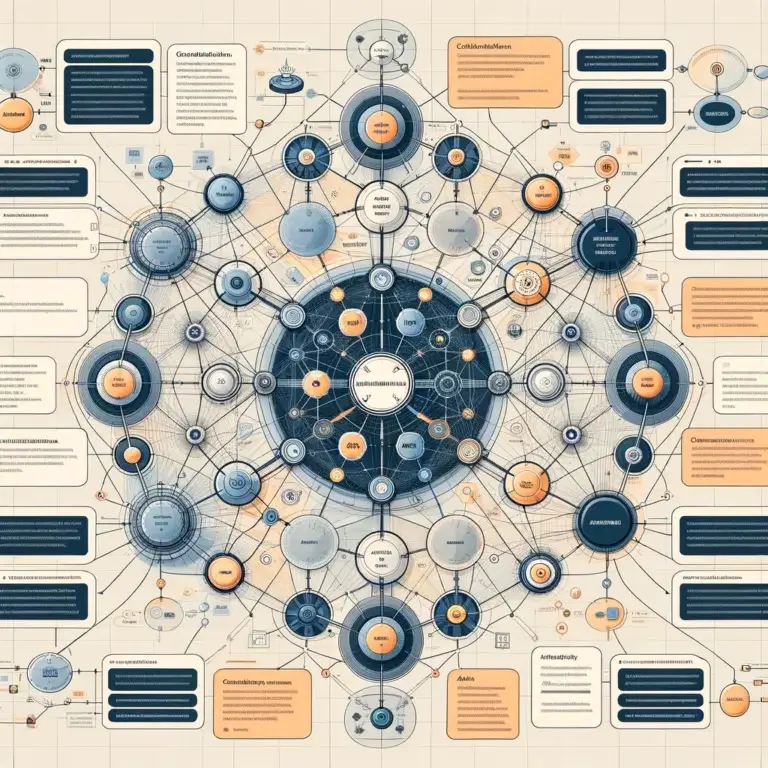
Almost everyone on the planet has been, is being, or will be affected somehow, by the latest version of Artificial Narrow Intelligence, known as Generative AI.
Whether it is the writer strikes that recently affected the Hollywood movie machine, the increasing popularity of ChatGPT in various business arenas, or emerging scandals caused by deep fake videos, no-one can deny that Generative AI is creating waves in today’s world.
Many have heard of it, yet few can describe exactly what it is. Nor can they differentiate between Generative AI and other forms of AI applications that have been influencing the world in the background for a good few years now.
A vast majority of AI focused researchers and programmers would probably claim the ultimate ambition of the AI industry is to create Artificial General Intelligence (AGI). AGI is effectively a human like consciousness that is capable of unrestricted creativity, self-awareness, and real time evaluation of unexpected events, amongst many other human-like traits.
Alas, their dreams are not likely to be realised any time soon. The current batch of Generative AI systems may well be able to mimic creativity in some form or another through the writing of articles, or the creation of pictures using language-based prompts but without additional internal retrospective and analytical algorithmic mechanisms, Generative AI will never become AGI.
Another area of AI research and development, that could be one of the keys to stepping closer to AGI, is that of Predictive AI. Predictive AI has become one of the mainstays for finance houses to control and operate monetary funds on the global markets.
Predictive AI and Generative AI are both basic forms of ANI that essentially use similar neural networks and Large Language Models (LLMs) to operate. Generative AI will use language analysis to work out what is being asked of it then access different databases of previously stored information and images before “creating” something resembling what has been asked of it. Predictive AI, in finance will use pattern recognition systems on data, both historical and real time, to recognise patterns in the data before “projecting/predicting” the next movements. Once these predictions are logged, some systems deliver them to human agents to act upon (Buy or Sell etc), while other systems are automated and will actively carry out required actions to create profit within the expected market movements (these systems are known as either High-Speed Trading Systems or Quant funds).
The emergence of organisations such as OpenAI, AI21 Labs, and Fifth Dimension AI etc’ has really increased awareness of the potential for generative AI as a disruptive technology for the world in general. The rise of predictive AI has been less publicised, but no less impactful, due to many of the top performing investment funds utilising some element of predictive AI in their analysis protocols.
As an example of generative AI in action, here are the bullet points that ChatGPT generated to highlight the differences between AI and MAS
Distinguishing Factors:
Simple AI vs. MAS AI Scope of Application:
Simple AI is tailored for specific tasks and excels within predefined domains. MAS AI is versatile and capable of addressing complex, multifaceted challenges that involve numerous interacting components. Learning and Adaptation:
Simple AI relies on structured data and predefined algorithms, with limited learning capabilities. MAS AI exhibits adaptive behaviour, with agents capable of learning from experiences and adjusting their strategies based on dynamic conditions. Autonomy and Collaboration:
Simple AI operates as a standalone system without collaboration between components. MAS AI relies on the autonomy of individual agents and their collaborative interactions to achieve collective objectives. Problem-Solving Approach:
Simple AI employs a focused problem-solving approach, suitable for well-defined tasks. MAS AI tackles complex problems by distributing tasks among autonomous agents, fostering collaboration to achieve overarching goals.
As AI has developed over the years, most systems have become more and more complex to the point where they are now considered to be Multi-Agent Systems (MAS). The terminology indicates that there are multiple separate, yet interacting, AI algorithms working to form or create the outputs the systems are designed for. Certainly, for Generative AI, the common systems seen today use various techniques such as pattern recognition, voice to text/text to speech, and access various libraries of text, statistics, and images etc’. Similarly, predictive AI is known to utilise pattern recognition, sentiment analysis, and chart analysis to indicate both short-term and long-term directional trends in the finance markets etc’.
MAS is the cornerstone of developing ANI, however it is nowhere near reaching the human like consciousness that is true AGI. The reason for this, in our opinion, is that most systems, in use today, are still exceptionally linear in their operations, with little to no flexibility of process or inbuilt recognition of the data passing through them.
To move forward, systems need to have an intrinsic ability to recognise important “glitches” or pertinent patterns before self-instructing to amend, research or update said findings. The creation of a self-cleansing data machine that is not just capable of churning through terabytes of data at a million miles an hour but is cognisant of potential changes in data patterns and able to ascertain the causes of such changes will be a major step forward in AIs potential for further complexity.
An example of this would be if an algorithm were tracking the historical movement of specific stock on the financial markets, it may recognise an overall increasing trend on the valuation but also a short-lived but deep decline during a 6-month period 5 years ago. Ordinarily, the algorithm would probably just ignore the decline as a glitch and focus on the overall trend.
A properly functioning MAS with the capability to recognise and research anomalies through alternative channels, not just the historical statistics, would perhaps find information correlating to the time in question and summarise that the decline was caused by something very specific (A fire at the facility, the chairman stepping down, strike action amongst workers etc’). It could then bring that information forward and be able to recognise potentially similar activities happening today and subsequently more accurately forecast current market movements.
Overall, it comes down to systems being able to look at both Micro and Macro perspectives within global markets, leveraging external data that is freely available through social media and other channels.
With regard to the overall strengthening of AIs complexity, Catena Cap have been steadily working on creating some complex MAS of its own. With a financial projection that utilises deception recognition in video presentations, contextual analysis of market movements, micro and macro news analysis protocols and a mechanism to spot, research, and act upon counter-trend behaviour.
We have also been looking at ways to expose the current trend of AI generated videos featuring politicians and celebrities either saying or doing something they wouldn’t ordinarily do IE Talking about fake investment opportunities or promoting the sale of different products. To provide a system through which, potentially, “Fake” videos can be analysed, Catena Cap has combined multiple systems including pixelation analysis, facial recognition, and voice recognition, along with many other AI-based analytical sub routines to form an overall system that can give an indication as to any specific video’s authenticity.
With a clear focus on leveraging AI to bring about serious business benefits, no stone has been left unturned regarding areas targeted for exploration. We have created and launched a plethora of MAS based services to better connect everyone to the benefits of high functioning AI.
Catena Cap is a privately owned organisation that operates within the closed/private hedge fund market. Utilizing a wide selection of internally created AI mechanisms and traditional analysis to guide its investment strategies Catena Cap sits in a rather unique position. Many of our customer facing services are, quite simply, integral parts of our main analytical system which have been given a user interface so as to be used individually while still performing their originally intended functions with the overall ecosystem.
Now, Catena Cap has brought its varied platforms forward so that consumers, corporations, enterprises and state-based organisations can engage with the world of AI and incorporate it into their daily lives and business. Using a combination of server and blockchain based data storage and retrieval systems, Catena Cap offers access to extensive data sets and data cleansing services across a varied selection of industries.
Some questions our systems can help answer:
As a subscription-based service provider, you are in control of the services you need. With standard packages available for individuals and bespoke packages available for larger corporations and enterprises. Some services offer a free trial period, or exposure to previous projections and results, so you can experience the benefits before taking a proper subscription.